The new AWS SDK for Kotlin with Coroutines support
The new AWS SDK for Kotlin was announced at AWS re:Invent in December 2021. Using the SDK, you can build Kotlin applications that work with Amazon S3, Amazon EC2, DynamoDB, and more. The SDK is currently in preview and is expected to become stable soon.
The SDK was designed from the ground up to support the Kotlin language and its best practices, providing Kotlin developers with a familiar and idiomatic experience for interacting with AWS. The SDK leverages Kotlin coroutines for asynchronous implementation, and there are plans to make it compatible with Kotlin multiplatform projects.

To start using the new SDK in your Kotlin programs, you’ll need to add a corresponding dependency to your project. For instance, if we would like to communicate with DynamoDB, then the aws.sdk.kotlin:dynamodb
module is required:
repositories { mavenCentral() } dependencies { implementation("org.jetbrains.kotlinx:kotlinx-coroutines-core:1.6.0") // The following line adds a dependency on the dynamodb client. // For demonstration purposes, we use 0.+ to get the latest version implementation("aws.sdk.kotlin:dynamodb:0.+") }
You can find the complete list of supported modules in the documentation for the AWS SDK.
After downloading the dependencies we can start using the API:
import kotlinx.coroutines.runBlocking import aws.sdk.kotlin.services.dynamodb.DynamoDbClient //sampleStart fun main() = runBlocking { val client = DynamoDbClient { region = "us-east-2" } val resp = client.listTables { limit = 10 } println("Current DynamoDB tables: ") resp.tableNames?.forEach { println(it) } client.close() } //sampleEnd
The program simply retrieves the list of tables and prints the table names into the standard output. In the example above, listTables
is a suspending function, so the code is wrapped into a runBlocking
call.
How about a slightly more complex example that demonstrates downloading every S3 object in a bucket to a local temporary directory, featuring paginated access, concurrent flows, and byte-streaming responses?
import aws.sdk.kotlin.services.s3.S3Client import aws.sdk.kotlin.services.s3.model.GetObjectRequest import aws.sdk.kotlin.services.s3.model.ListObjectsV2Request import aws.sdk.kotlin.services.s3.paginators.listObjectsV2Paginated import aws.smithy.kotlin.runtime.content.writeToFile import kotlinx.coroutines.flow.asFlow import kotlinx.coroutines.flow.filter import kotlinx.coroutines.flow.flatMapConcat import kotlinx.coroutines.flow.flowOf import java.nio.file.Paths import kotlin.io.path.createDirectories //sampleStart suspend fun downloadAllS3Objects(bucketName: String) { val s3 = S3Client.fromEnvironment() val listReq = ListObjectsV2Request { bucket = bucketName } s3.listObjectsV2Paginated(listReq) .flatMapConcat { it.contents?.asFlow() ?: flowOf() } .filter { it.size > 0 } .collect { obj -> val getReq = GetObjectRequest { bucket = bucketName key = obj.key } s3.getObject(getReq) { val path = Paths.get(System.getProperty("java.io.tmpdir"), obj.key) path.parent.createDirectories() it.body?.writeToFile(path) } } } //sampleEnd
In the example above, you can see again the use of the suspending functions in the SDK: the getObject
and writeToFile
functions are all marked with the suspend
keyword.
In the official documentation for the new AWS SDK for Kotlin, you can find detailed step-by-step instructions on how to get started. Also, you can find a number of interesting examples that demonstrate the API with a number of AWS services such as Amazon DynamoDB, S3, Rekognition, Amazon Simple Notification Service, and AWS Key Management Service.
The new AWS SDK for Kotlin is in active development and you can check out what features are planned in the roadmap. Try it now and let us know what you think!
The new AWS SDK for Kotlin with Coroutines support
The new AWS SDK for Kotlin was announced at AWS re:Invent in December 2021. Using the SDK, you can build Kotlin applications that work with Amazon S3, Amazon EC2, DynamoDB, and more. The SDK is currently in preview and is expected to become stable soon.
The SDK was designed from the ground up to support the Kotlin language and its best practices, providing Kotlin developers with a familiar and idiomatic experience for interacting with AWS. The SDK leverages Kotlin coroutines for asynchronous implementation, and there are plans to make it compatible with Kotlin multiplatform projects.

To start using the new SDK in your Kotlin programs, you’ll need to add a corresponding dependency to your project. For instance, if we would like to communicate with DynamoDB, then the aws.sdk.kotlin:dynamodb
module is required:
repositories { mavenCentral() } dependencies { implementation("org.jetbrains.kotlinx:kotlinx-coroutines-core:1.6.0") // The following line adds a dependency on the dynamodb client. // For demonstration purposes, we use 0.+ to get the latest version implementation("aws.sdk.kotlin:dynamodb:0.+") }
You can find the complete list of supported modules in the documentation for the AWS SDK.
After downloading the dependencies we can start using the API:
import kotlinx.coroutines.runBlocking import aws.sdk.kotlin.services.dynamodb.DynamoDbClient //sampleStart fun main() = runBlocking { val client = DynamoDbClient { region = "us-east-2" } val resp = client.listTables { limit = 10 } println("Current DynamoDB tables: ") resp.tableNames?.forEach { println(it) } client.close() } //sampleEnd
The program simply retrieves the list of tables and prints the table names into the standard output. In the example above, listTables
is a suspending function, so the code is wrapped into a runBlocking
call.
How about a slightly more complex example that demonstrates downloading every S3 object in a bucket to a local temporary directory, featuring paginated access, concurrent flows, and byte-streaming responses?
import aws.sdk.kotlin.services.s3.S3Client import aws.sdk.kotlin.services.s3.model.GetObjectRequest import aws.sdk.kotlin.services.s3.model.ListObjectsV2Request import aws.sdk.kotlin.services.s3.paginators.listObjectsV2Paginated import aws.smithy.kotlin.runtime.content.writeToFile import kotlinx.coroutines.flow.asFlow import kotlinx.coroutines.flow.filter import kotlinx.coroutines.flow.flatMapConcat import kotlinx.coroutines.flow.flowOf import java.nio.file.Paths import kotlin.io.path.createDirectories //sampleStart suspend fun downloadAllS3Objects(bucketName: String) { val s3 = S3Client.fromEnvironment() val listReq = ListObjectsV2Request { bucket = bucketName } s3.listObjectsV2Paginated(listReq) .flatMapConcat { it.contents?.asFlow() ?: flowOf() } .filter { it.size > 0 } .collect { obj -> val getReq = GetObjectRequest { bucket = bucketName key = obj.key } s3.getObject(getReq) { val path = Paths.get(System.getProperty("java.io.tmpdir"), obj.key) path.parent.createDirectories() it.body?.writeToFile(path) } } } //sampleEnd
In the example above, you can see again the use of the suspending functions in the SDK: the getObject
and writeToFile
functions are all marked with the suspend
keyword.
In the official documentation for the new AWS SDK for Kotlin, you can find detailed step-by-step instructions on how to get started. Also, you can find a number of interesting examples that demonstrate the API with a number of AWS services such as Amazon DynamoDB, S3, Rekognition, Amazon Simple Notification Service, and AWS Key Management Service.
The new AWS SDK for Kotlin is in active development and you can check out what features are planned in the roadmap. Try it now and let us know what you think!
适用于 Kotlin 并支持协同程序的新 AWS SDK
适用于 Kotlin 的新 AWS SDK 已于 2021 年 12 月在 AWS re:Invent 上宣布。 您可以使用此 SDK 构建与 Amazon S3、Amazon EC2、DynamoDB 等协同工作的 Kotlin 应用程序。 SDK 目前处于预览阶段,预计很快会推出稳定版。
从头开始设计 SDK 是为了支持 Kotlin 语言及其最佳做法,为 Kotlin 开发者提供与 AWS 交互的熟悉和惯用体验。 SDK 利用 Kotlin 协同程序进行异步实现,并计划使其兼容 Kotlin 多平台项目。
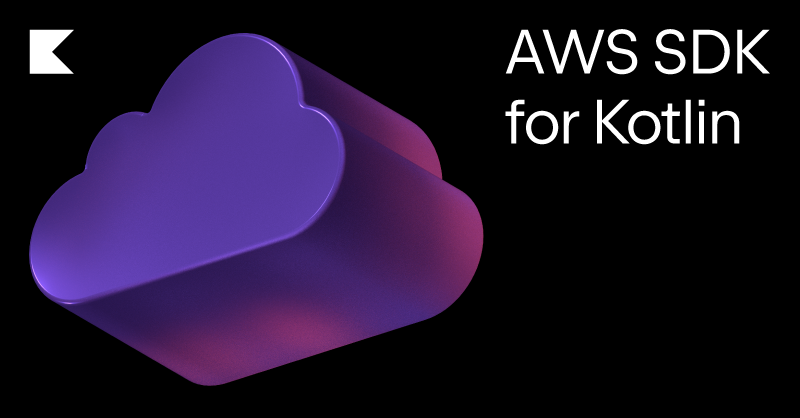
要开始在 Kotlin 程序中使用新 SDK,您需要向项目添加相应的依赖项。 例如,如果要与 DynamoDB 通信,则需要 aws.sdk.kotlin:dynamodb
模块:
repositories { mavenCentral() } dependencies { implementation("org.jetbrains.kotlinx:kotlinx-coroutines-core:1.6.0") // The following line adds a dependency on the dynamodb client. // For demonstration purposes, we use 0.+ to get the latest version implementation("aws.sdk.kotlin:dynamodb:0.+") }
您可以在 AWS SDK 文档中找到受支持模块的完整列表。
下载依赖项后,即可开始使用 API:
import kotlinx.coroutines.runBlocking import aws.sdk.kotlin.services.dynamodb.DynamoDbClient //sampleStart fun main() = runBlocking { val client = DynamoDbClient { region = "us-east-2" } val resp = client.listTables { limit = 10 } println("Current DynamoDB tables: ") resp.tableNames?.forEach { println(it) } client.close() } //sampleEnd
该程序只会检索表的列表,并将表名打印到标准输出中。 在上面的示例中,listTables
是一个挂起函数,因此代码被包装到 runBlocking
调用中。
我们来看一个稍微复杂一点的示例,演示如何将存储桶中的每个 S3 对象下载到本地临时目录(包含分页访问、并发流和字节流响应)。
import aws.sdk.kotlin.services.s3.S3Client import aws.sdk.kotlin.services.s3.model.GetObjectRequest import aws.sdk.kotlin.services.s3.model.ListObjectsV2Request import aws.sdk.kotlin.services.s3.paginators.listObjectsV2Paginated import aws.smithy.kotlin.runtime.content.writeToFile import kotlinx.coroutines.flow.asFlow import kotlinx.coroutines.flow.filter import kotlinx.coroutines.flow.flatMapConcat import kotlinx.coroutines.flow.flowOf import java.nio.file.Paths import kotlin.io.path.createDirectories //sampleStart suspend fun downloadAllS3Objects(bucketName: String) { val s3 = S3Client.fromEnvironment() val listReq = ListObjectsV2Request { bucket = bucketName } s3.listObjectsV2Paginated(listReq) .flatMapConcat { it.contents?.asFlow() ?: flowOf() } .filter { it.size > 0 } .collect { obj -> val getReq = GetObjectRequest { bucket = bucketName key = obj.key } s3.getObject(getReq) { val path = Paths.get(System.getProperty("java.io.tmpdir"), obj.key) path.parent.createDirectories() it.body?.writeToFile(path) } } } //sampleEnd
在上面的示例中,您可以再次看到 SDK 中挂起函数的使用:getObject
和 writeToFile
函数都使用 suspend
关键字进行标记。
您可以在适用于 Kotlin 的新 AWS SDK 的官方文档中找到有关如何入门的详细分步说明。 此外,您还可以找到许多有趣的示例,它们使用 Amazon DynamoDB、S3、Rekognition、Amazon Simple Notification Service 和 AWS Key Management Service 等一系列 AWS 服务来演示 API。
适用于 Kotlin 的新 AWS SDK 正在积极开发中,您可以在路线图中查看计划的功能。 立即试用,并告诉我们您的想法!
英文博文原作者:
Introducing kotlinx.coroutines 1.6.0
Following the release of Kotlin 1.6.0, the 1.6.0 version of the kotlinx.coroutines
library is out. Here are the main features it brings:
- A new API and multiplatform support for
kotlinx-coroutines-test
introduce a common solution for writing portable tests with suspending functions. - Support for the new Kotlin/Native memory manager makes multithreaded coroutines for Kotlin/Native available out of the box.
- The new dispatcher views API lets you limit parallelism without creating additional thread pools and provides a unified way to create dispatchers for unbound parallelism.
- The introduction of
CopyableThreadContextElement
gives coroutines thread-safe write access toThreadLocal
variables. - Migration to the Java 8 target: we’ve taken the first step of this migration by compiling against Java 8 source and binary targets.
In this blog post, we’ll take a closer look at all the new features. To try them out right away, jump to the How to try it section.
A new API and multiplatform support for kotlinx-coroutines-test
Following our roadmap, we completely reworked kotlinx-coroutines-test
. The testing module received multiplatform support and solved the problem of writing portable tests with suspending functions, which we decided to shift into the library space. The new experimental API also fixed multiple issues with the previously used runBlockingTest()
scheme.
The entry point to the new API is the runTest()
function, which you can use on any platform to test code that involves coroutines. runTest()
will automatically skip calls to delay()
and handle uncaught exceptions. Unlike runBlockingTest()
, it will wait for asynchronous callbacks to handle situations where some code runs in dispatchers that are not integrated with the test module.
runTest()
only once per test and immediately return its result. This restriction is necessary for the test to work on the JS platform. If for some reason you need to call runTest()
several times, please share your use case using the issue tracker.
@Test fun testF() = runTest { // Run a coroutine with virtual time val actual = f() val expected = 42 assertEquals(actual, expected) } suspend fun f(): Int { delay(1_000) // Will finish immediately instead of delaying return 42 }
You can find a detailed description of the API in the module’s README. To adapt the existing test code to the new scheme, please follow the migration guide. The old API has now been deprecated.
Support for the new Kotlin/Native memory manager
The GitHub issue about supporting multithreaded coroutines for Kotlin/Native has received a huge number of upvotes. In 1.3.2, we started maintaining a companion library version that included the feature’s experimental implementation within the regular Kotlin/Native memory model. Since then, we have started publishing a separate native-mt
artifact for each release to share this implementation.
With Kotlin 1.6.0, we announced the new experimental Kotlin/Native memory management scheme, which made the limitations of the existing native-mt
version possible to overcome. In this release, we implemented support for the new memory manager and merged the implementation into the mainline. This means you only need the 1.6.0 version of kotlinx.coroutines
to try experimental Kotlin/Native multithreading with the new memory model.
Since the old native-mt
implementation still suffers from memory leaks in some concurrent execution scenarios, we are going to decommission it starting with kotlinx.coroutines
1.7.0. For the 1.6.* releases, native-mt
artifacts will still be published. You can migrate to the new memory management scheme by following the migration guide.
Dispatcher views API
You might want to control concurrency while using coroutines, for example, to limit the number of concurrent requests to a database. A popular solution for this is to define a custom coroutine dispatcher with the newFixedThreadPoolContext() function which is then used on every database invocation:
class UserRepository { private val DB = newFixedThreadPoolContext(10, "DB") suspend fun getUserById(userId: Int): User? = withContext(DB) { executeQuery("SELECT * FROM users WHERE id = $1", userId).singleOrNull() } }
Unfortunately, this approach has several problems:
newFixedThreadPoolContext()
can create many unnecessary threads, most of which are idle, consuming the memory, CPU, and device battery.- Every
withContext(DB)
invocation performs an actual switch to a different thread, which can be extremely resource intensive. - The result of
newFixedThreadPoolContext()
needs to be explicitly closed when no longer used. This is quite error-prone, often forgotten about, and can lead to leaking threads. - If you have several thread pools as separate executors, they cannot share threads and resources.
In kotlinx.coroutines
1.6.0, we’ve introduced the new dispatcher views API as an option to limit concurrency without creating additional threads and allocating extra resources. To start using dispatcher views, just call limitedParallelism()
instead of newFixedThreadPoolContext()
.
class UserRepository { private val DB = Dispatchers.IO.limitedParallelism(10) suspend fun getUserById(userId: Int): User? = withContext(DB) { executeQuery("SELECT * FROM users WHERE id = $1", userId).singleOrNull() } }
The new approach addresses the limitations of using custom thread pools:
- A dispatcher view is only a wrapper to the original dispatcher. Using the original dispatcher’s resources, it limits the number of coroutines that can be executed simultaneously and doesn’t create new threads.
- The
withContext()
invocation withDispatchers.Default
,Dispatchers.IO
, or their views attempts not to switch threads when possible. - A view doesn’t need to be closed.
- To create separate executors, you can take multiple views of the same dispatcher and they will share threads and resources. There is no limit on the total parallelism value, but the effective parallelism of all views cannot exceed the actual parallelism of the original dispatcher. This means that you can control the parallelism of both the entire application and each view separately.
val backgroundDispatcher = newFixedThreadPoolContext(5, "App Background") // At most 2 threads will process images val imageProcessingDispatcher = backgroundDispatcher.limitedParallelism(2) // At most 1 thread will do IO val fileWriterDispatcher = backgroundDispatcher.limitedParallelism(1) // At most 3 threads will handle database requests val dbDispatcher = backgroundDispatcher.limitedParallelism(3) // At most 5 coroutines can be executed simultaneously
Dispatchers.IO
elasticity for limited parallelism
Imagine a case where your application uses multiple views as separate executors and needs each one to guarantee a specified level of parallelism during peak loads. You don’t need to create a parent dispatcher with the desired total parallelism value. Instead, you can use views of Dispatchers.IO
which can create and shutdown additional threads on-demand, saving resources in a steady state.
The implementation of limitedParallelism()
for Dispatchers.IO
is elastic. This means that Dispatchers.IO
itself still has a limit of 64 threads, but each of its views will have the effective parallelism of the requested value.
// 80 threads for PostgreSQL connection val myPostgresqlDbDispatcher = Dispatchers.IO.limitedParallelism(80) // 40 threads for MongoDB connection val myMongoDbDispatcher = Dispatchers.IO.limitedParallelism(40)
In the example:
- During peak loads, the system may have up to 64 + 80 + 40 threads dedicated to blocking tasks.
- In a steady state, there will be only a small number of threads shared among
Dispatchers.IO
,myPostgresqlDbDispatcher
, andmyMongoDbDispatcher
.
Under the hood, it works with an additional dispatcher backed by an unlimited pool of threads. Both Dispatchers.IO
and its views are actually views of that dispatcher and share threads and resources.
Introduction of CopyableThreadContextElement
In Java, you can use a ThreadLocal
variable to maintain some value related to the current thread. In kotlinx.coroutines
, the same can be achieved with ThreadContextElement
. However, since ThreadContextElement
is a part of CoroutineContext
, it gets inherited by child coroutines, which can execute concurrently. This can be a problem if the underlying value is not thread-safe and gets concurrently mutated, for example, when implementing logging contexts or tracing frameworks.
To resolve the issue, we created the new CopyableThreadContextElement
interface, which defines an extra copyForChildCoroutine()
method. During the child coroutine’s launch time, the method will be called on the parent ThreadContextElement
instance to obtain a new ThreadContextElement
for the child coroutine’s context. Thus, every coroutine will have its own element copy that it can mutate, guaranteeing thread safety for the underlying value.
Migration to the Java 8 target
Maintaining support for the Java 6 target requires a lot of work and prevents us from using potentially helpful features that Java 8 offers. Kotlin already migrated to Java 8 in version 1.5.0. Catching up with the language, kotlinx.coroutines
1.6.0 has begun the migration process by compiling against the Java 8 source and binary targets.
Although support for the Java 6 target has been dropped, kotlinx.coroutines
1.6.0 remains compatible with popular Android API levels and uses new features of Java 7+ only if there is a way to provide a proper fallback.
How to try it
kotlinx.coroutines
1.6.0 is available from Maven Central. To try it in your project, do the following:
- Make sure that you have
mavenCentral()
in the list of repositories:
repository { mavenCentral() }
- Make sure that you are using the latest version of Kotlin:
plugins { kotlin("jvm") version "1.6.0" }
- Add
kotlinx.coroutines
as a dependency:
dependencies { implementation("org.jetbrains.kotlinx:kotlinx-coroutines-core:1.6.0") }
If you run into any trouble
- Report issues to the GitHub issue tracker.
- Look for help in the #coroutines channel on the Kotlin Slack (get an invite).
Read more
Concurrent Coroutines – Concurrency is not Parallelism
On Kotlin Coroutines and how concurrency is different from parallelism
The official docs describe Kotlin Coroutines as a tool “for asynchronous programming and more”, especially are coroutines supposed to support us with “asynchronous or non-blocking programming”. What exactly does this mean? How is “asynchrony” related to the terms “concurrency” and “parallelism”, tags we hear about a lot in this context as well. In this article, we will see that coroutines are mostly concerned about concurrency and not primarily about parallelism. Coroutines provide sophisticated means which help us structure code to make it highly concurrently executable, also enabling parallelism, which isn’t the default behavior though. If you don’t understand the difference yet, don’t worry about it, it will get clearer throughout the article. Many people, I included, struggle to make use of these terms correctly. Let’s learn more about coroutines and how they relate to the discussed topics.
(You can find a general introduction to Kotlin coroutines in this article)
Asynchrony – A programming model
Asynchronous programming is a topic we’ve been reading and hearing about a lot in the last couple of years. It mainly refers to “the occurrence of events independent of the main program flow” and also “ways to deal with these events” (Wikipedia). One crucial aspect of asynchronous programming is the fact that asynchronously started actions do not immediately block the program and take place concurrently. When programming asynchronously, we often find ourselves triggering some subroutine that immediately returns to the caller to let the main program flow continue without waiting for the subroutine’s result. Once the result is needed, you may run into two scenarios: 1) the result has been fully processed and can just be requested or 2) You need to block your program until it is available. That is how futures or promises work. Another popular example of asynchrony is how reactive streams work like as described in the Reactive Manifesto:
Reactive Systems rely on asynchronous message-passing to establish a boundary between components that ensures loose coupling, isolation and location transparency. […] Non-blocking communication allows recipients to only consume resources while active, leading to less system overhead.
Altogether, we can describe asynchrony, defined in the domain of software engineering, as a programming model that enables non-blocking and concurrent programming. We dispatch tasks to let our program continue doing something else until we receive a signal that the results are available. The following image illustrated this:
We want to continue reading a book and therefore let a machine do the washing for us.
Disclaimer: I took this and also the two following images from this Quora post which also describes the discussed terms.
Concurrency – It’s about structure
After we learned what asynchrony refers to, let’s see what concurrency is. Concurrency is not, as many people mistakenly believe, about running things “in parallel” or “at the same time”. Rob Pike, a Google engineer, best known for his work on Go, describes concurrency as a “composition of independently executing tasks” and he emphasizes that concurrency really is about structuring a program. That means that a concurrent program handles multiple tasks being in progress at the same time but not necessarily being executed simultaneously. The work on all tasks may be interleaved in some arbitrary order, as nicely illustrated in this little image:
Concurrency is not parallelism. It tries to break down tasks which we don’t necessarily need to execute at the same time. Its primary goal is structure, not parallelism.
Parallelism – It’s about execution
Parallelism, often mistakenly used synonymously for concurrency, is about the simultaneous execution of multiple things. If concurrency is about structure, then parallelism is about the execution of multiple tasks. We can say that concurrency makes the use of parallelism easier, but it is not even a prerequisite since we can have parallelism without concurrency.
Conclusively, as Rob Pike describes it: “Concurrency is about dealing with lots of things at once. Parallelism is about doing lots of things at once”. You can watch his talk “Concurrency is not Parallelism” on YouTube.
Coroutines in terms of concurrency and parallelism
Coroutines are about concurrency first of all. They provide great tools that let us break down tasks into various chunks which are not executed simultaneously by default. A simple example illustrating this is part of the Kotlin coroutines documentation:
fun main() = runBlocking<Unit> {
val time = measureTimeMillis {
val one = async { doSomethingUsefulOne() }
val two = async { doSomethingUsefulTwo() }
println("The answer is ${one.await() + two.await()}")
}
println("Completed in $time ms")
}
suspend fun doSomethingUsefulOne(): Int {
delay(1000L)
return 13
}
suspend fun doSomethingUsefulTwo(): Int {
delay(1000L)
return 29
}
The example terminates in roughly 1000 milliseconds since both “somethingUseful” tasks take about 1 second each and we execute them asynchronously with the help of the async
coroutine builder. Both tasks just use a simple non-blocking delay
to simulate some reasonably long-running action. Let’s see if the framework executes these tasks truly simultaneously. Therefore we add some log statements that tell us the threads the actions run on:
[main] DEBUG logger - in runBlocking
[main] DEBUG logger - in doSomethingUsefulOne
[main] DEBUG logger - in doSomethingUsefulTwo
Since we use runBlocking
from the main thread, it also runs on this one. The async
builders do not specify a separate CoroutineScope or CoroutineContext and therefore also inherently run on main
.
We have two tasks run on the same thread, and they finish after a 1-second delay. That is possible since delay
only suspends the coroutine and does not block main
. The example is, as correctly described, an example of concurrency, not utilizing parallelism. Let’s change the functions to something that really takes its time and see what happens.
Parallel Coroutines
Instead of just delaying the coroutines, we let the functions doSomethingUseful
calculate the next probable prime based on a randomly generated BigInteger
which happens to be a fairly expensive task (since this calculation is based on a random it will not run in deterministic time):
fun doSomethingUsefulOne(): BigInteger {
log.debug("in doSomethingUsefulOne")
return BigInteger(1500, Random()).nextProbablePrime()
}
Note that the suspend
keyword is not necessary anymore and would actually be misleading. The function does not make use of other suspending functions and blocks the calling thread for the needed time. Running the code results in the following logs:
22:22:04.716 [main] DEBUG logger - in runBlocking
22:22:04.749 [main] DEBUG logger - in doSomethingUsefulOne
22:22:05.595 [main] DEBUG logger - Prime calculation took 844 ms
22:22:05.602 [main] DEBUG logger - in doSomethingUsefulOne
22:22:08.241 [main] DEBUG logger - Prime calculation took 2638 ms
Completed in 3520 ms
As we can easily see, the tasks still run concurrently as in with async
coroutines but don’t execute at the same time anymore. The overall runtime is the sum of both sub-calculations (roughly). After changing the suspending code to blocking code, the result changes and we don’t win any time while execution anymore.
Note on the example
Let me note that I find the example provided in the documentation slightly misleading as it concludes with “This is twice as fast, because we have concurrent execution of two coroutines” after applying async
coroutine builders to the previously sequentially executed code. It only is “twice as fast” since the concurrently executed coroutines just delay in a non-blocking way. The example gives the impression that we get “parallelism” for free although it’s only meant to demonstrate asynchronous programming as I see it.
Now how can we make coroutines run in parallel? To fix our prime example from above, we need to dispatch these tasks on some worker threads to not block the main thread anymore. We have a few possibilities to make this work.
Making coroutines run in parallel
1. Run in GlobalScope
We can spawn a coroutine in the GlobalScope
. That means that the coroutine is not bound to any Job
and only limited by the lifetime of the whole application. That is the behavior we know from spawning new threads. It’s hard to keep track of global coroutines, and the whole approach seems naive and error-prone. Nonetheless, running in this global scope dispatches a coroutine onto Dispatchers.Default
, a shared thread pool managed by the kotlinx.coroutines library. By default, the maximal number of threads used by this dispatcher is equal to the number of available CPU cores, but is at least two.
Applying this approach to our example is simple. Instead of running async
in the scope of runBlocking
, i.e., on the main thread, we spawn them in GlobalScope
:
val time = measureTimeMillis {
val one = GlobalScope.async { doSomethingUsefulOne() }
val two = GlobalScope.async { doSomethingUsefulTwo() }
}
The output verifies that we now run in roughly max(time(calc1), time(calc2))
:
22:42:19.375 [main] DEBUG logger - in runBlocking
22:42:19.393 [DefaultDispatcher-worker-1] DEBUG logger - in doSomethingUsefulOne
22:42:19.408 [DefaultDispatcher-worker-4] DEBUG logger - in doSomethingUsefulOne
22:42:22.640 [DefaultDispatcher-worker-1] DEBUG logger - Prime calculation took 3245 ms
22:42:23.330 [DefaultDispatcher-worker-4] DEBUG logger - Prime calculation took 3922 ms
Completed in 3950 ms
We successfully applied parallelism to our concurrent example. As I said though, this fix is naive and can be improved further.
2. Specify a coroutine dispatcher
Instead of spawning async
in the GlobalScope
, we can still let them run in the scope of, i.e., as a child of, runBlocking
. To get the same result, we explicitly set a coroutine dispatcher now:
val time = measureTimeMillis {
val one = async(Dispatchers.Default) { doSomethingUsefulOne() }
val two = async(Dispatchers.Default) { doSomethingUsefulTwo() }
println("The answer is ${one.await() + two.await()}")
}
This adjustment leads to the same result as before while not losing the child-parent structure we want. We can still do better though. Wouldn’t it be most desirable to have real suspending functions again? Instead of taking care of not blocking the main thread while executing blocking functions, it would be best only to call suspending functions that don’t block the caller.
3. Make blocking function suspending
We can use withContext
which “immediately applies dispatcher from the new context, shifting execution of the block into the different thread inside the block, and back when it completes”:
suspend fun doSomethingUsefulOne(): BigInteger = withContext(Dispatchers.Default) {
executeAndMeasureTimeMillis {
log.debug("in doSomethingUsefulOne")
BigInteger(1500, Random()).nextProbablePrime()
}
}.also {
log.debug("Prime calculation took ${it.second} ms")
}.first
With this approach, we confine the execution of dispatched tasks to the prime calculation inside the suspending function. The output nicely demonstrates that only the actual prime calculation happens on a different thread while everything else stays on main. When has multi-threading ever been that easy? I really like this solution the most.
(The function executeAndMeasureTimeMillis
is a custom one that measures execution time and returns a pair of result and execution time)
23:00:20.591 [main] DEBUG logger - in runBlocking
23:00:20.648 [DefaultDispatcher-worker-1] DEBUG logger - in doSomethingUsefulOne
23:00:20.714 [DefaultDispatcher-worker-2] DEBUG logger - in doSomethingUsefulOne
23:00:21.132 [main] DEBUG logger - Prime calculation took 413 ms
23:00:23.971 [main] DEBUG logger - Prime calculation took 3322 ms
Completed in 3371 ms
Caution: We use Concurrency and Parallelism interchangeably although we should not
As already mentioned in the introductory part of this article, we often use the terms parallelism and concurrency as synonyms of each other. I want to show you that even the Kotlin documentation does not clearly differentiate between both terms. The section on “Shared mutable state and concurrency” (as of 11/5/2018, may be changed in future) introduces with:
Coroutines can be executed concurrently using a multi-threaded dispatcher like the
Dispatchers.Default
. It presents all the usual concurrency problems. The main problem being synchronization of access to shared mutable state. Some solutions to this problem in the land of coroutines are similar to the solutions in the multi-threaded world, but others are unique.
This sentence should really read “Coroutines can be executed in parallel using multi-threaded dispatchers like Dispatchers.Default
…”
Conclusion
It’s important to know the difference between concurrency and parallelism. We learned that concurrency is mainly about dealing with many things at once while parallelism is about executing many things at once. Coroutines provide sophisticated tools to enable concurrency but don’t give us parallelism for free. In some situations, it will be necessary to dispatch blocking code onto some worker threads to let the main program flow continue. Please remember that we mostly need parallelism for CPU intensive and performance critical tasks. In most scenarios, it might be just fine to don’t worry about parallelism and be happy about the fantastic concurrency we get from coroutines.
Lastly, let me say Thank you to Roman Elizarov who discussed these topics with me before I wrote the article. 🙏🏼
The post Concurrent Coroutines – Concurrency is not Parallelism appeared first on Kotlin Expertise Blog.